A dual diagnosis (substance related disorder combined with other clinical and/or personality disorders) is associated with a broad range of adverse outcomes, such as higher suicide risks and criminality. The present study is the first large-scale study aimed at comparing adult violent offenders with a Dual Diagnosis (DD-group, n=710) with other subgroups of adult violent offenders: offenders without a clinical or personality disorder (No disorders group, n=142); offenders being diagnosed with only Substance Use Disorders (SUD-group, n=120); Offenders with other clinical (SUDs excluded) and/or personality disorders (Other disorders group, n=499). Seven of the central disorders (Other disorders group, n=499). Seven of the central eight criminogenic risk factors (categorized in the big and moderate three criminogenic risk factors) described in General Personality and Cognitive Social Learning (GPCSL) theories were included in a multi-group path analysis as predictors of general recidivism and dropout. DD-offenders had the highest recidivism and dropout rates. A few numbers of the central eight risk factors were found predictive of general recidivism and dropout within each subgroup. In DD-offenders the only risk factor relevant for recidivism was a history of antisocial/criminal behavior.
The literature suggests that adults with a dual diagnosis have an increased risk for delinquent behavior compared to the general population [1,2]. A Dual Diagnosis (DD) is defined by the World Health Organization as the simultaneous manifestation of a Substance Use Disorder (SUD) and another clinical and/or personality disorder within one and the same patient [3]. Empirical studies among adult offenders report on percentages varying from 7.4% [4] to 26.3% [5,6] of prisoners with a dual diagnosis, depending-amongst others-on a more narrow or broad definition of a dual diagnosis. While prevalence rates of adult offenders diagnosed with exclusively substance use disorders (52.3%) were higher offenders, with a dual diagnosis recidivated at a higher rate [4]. Walter, Wiesbeck, Dittmann, and Graf [7], for instance, investigated a sample of 379 offenders who were subject to court orders for forensic psychiatric evaluation. In a median time at risk of 8.4 years, the highest general recidivism rate (69.3%) was found among DD-offenders against 44.6% in the subgroup of offenders with a Substance Use Disorder (SUD). They also recidivated more than offenders with a personality disorder (33%) and other diagnoses (25.2%). Similar findings were presented by Lund, Van der Hof, Forsman, Anckarsäter, and Nilsson [8] who studied a population-based cohort of 349 offenders with a Personality Disorder (PD) sentenced to forensic psychiatric treatment, prison, or noncustodial sanctions. Survival analyses were used to compare recidivism rates across three different sanctioned and four diagnostic groups (PD-group, SUD-group, DD-group, residual disorder group). The course of violent recidivism did not differ between the sanctioned groups; however, they did find significant differences between the diagnostic groups. The shortest survival time and the highest rate of violent recidivism were found in the DD-group and the SUD-group (respectively 58% and 56% versus 43% in the PD-group, 30% in the residual group). Apart from an increased risk for criminal behavior, dually diagnosed adults also have a higher risk of morbidity and mortality, especially death by unnatural causes, compared to the general population [9], with a shorter life expectancy of 15 to 20 years [10]. In a Danish nation-wide population-based study, the prevalence of SUDs in adults with mental disorders was found to be 30.4% [11]. The more often visited first aid posts and hospital emergency rooms than people without SUDs. Moreover, hospitalization and use of outpatient treatment contacts was found to be inconsistent and discontinuous among DD-adults. Factors such as unstable housing, deficits in adequate coping skills, and problematic interpersonal relationships are assumed to act as barriers for accessing medical and social services [11], while they are in need of long-term (clinical) care, but at the same time are not very responsive to treatment. Therapeutic relations are difficult to establish, dropout rates are high and treatment prognoses are bad [12,13]. Clinical predictors of dropout from substance user treatment include early onset of substance use, impaired coping, and lower social support [14,15]. From the previous it can be concluded that dually diagnosed adults are at a high risk for reoffending in particular due to the presence of a number of critical risk factors such as unstable relationships and inadequate problem solving skills. Amongst others, these factors are assumed to lie at the core of the development of criminal conduct as described in the General Personality and Cognitive Social Learning (GPCSL) theory and its related Risk-Need-Responsivity (RNR) model, a rehabilitation model for the assessment and treatment of offenders [16]. The present study sets out to - based on the GPCSL-theory-identify the risk factors for reoffending (recidivism) among dually diagnosed violent offenders in outpatient forensic care. To our knowledge this is the first large-scale study among forensic outpatient violent offenders in which the RNR-based risk factors are related to adverse outcomes (i.e., general recidivism and dropout).
The General Personality and Cognitive Social Learning (GPCSL) theory is a general theory of the onset and sustained criminal behavior [16]. In the GPCSL, specific criminogenic variables within the individual and his/her social learning environment are described that set this theory apart from mainstream criminological theories and clinical perspectives of criminal behaviour. For instance, the traditional clinical variables of anxiety, depression, mood, and major psychotic symptoms, are salient from a clinical perspective of criminal behaviour, however, in GPCSL, such variables are regarded as minor risk factors. A meta-analytic study conducted by Bonta, Blais, and Wilson [17] confirmed that, with the exception of antisocial personality, the clinical variables were not predictive of recidivism.
GPCSL recognizes that there are many routes to crime but some experiences in life are more influential than others. For example, the lack of employment, poor use of leisure time, substance abuse and having a criminal social network have a far greater impact on the likelihood of criminal behaviour relative to one’s socioeconomic conditions. Such situations limit exposure to rewards for prosocial behaviour and also diminish punishment for rule violating behaviour. The GPCSL theoretical perspective views the following predominantly individual variables as major determinants of criminality: History of antisocial/criminal behavior, Antisocial Personality Pattern, Antisocial Cognitions, and Antisocial network. They are referred to as the big four criminogenic risk factors and are assumed to facilitate the commission of a criminal act. Within GPCSL, another set of predominantly contextual domains are described as relevant in the development of criminal behaviour. These are: Education/Employment, Family/Marital, Substance Abuse, and Leisure pursuits and are referred as the moderate four criminogenic risk factors. Together, the big and moderate four criminogenic risk factors go under the name central eight criminogenic risk factors.
A number of meta-analytic reviews have found evidence for the predictive validity of the central eight criminogenic factors and for the primacy of the big four over the moderate four risk factors among general offenders [18-20]. Moreover, the central eight risk factors were found relevant across age [21,22] gender [23-25] ethnic groups [26] and offender types [27,28]. Although the evidence supports the central eight risk factors as being applicable to a range of offenders, results are inconsistent with regard to the primacy of the big four over the moderate four risk factors among these offender subgroups. Further research is needed to strengthen the applicability of the central eight to other offender subgroups in general and to find support for the greater importance of the big four criminogenic factors over the moderate four in particular to reduce the risk of reoffending through treatment [17].
One of the most influential models in guiding treatment interventions with offenders is the Risk-Need-Responsivity (RNR) model [29,30]. Of the 15 principles currently represented in the model [16], three of them have been at the core since 1990. These are: Risk-principle that specify that the intensity and duration of the treatment should match the offender’s risk of re-offending; need-principle dictates that treatment should target the dynamic (changeable) criminogenic risk factors; responsivity-principle that describes that treatment should be responsive to specific offender’s abilities (e.g., intellectual capacities and motivation) to maximize treatment engagement. In their most recent review Andrews and Bonta [16] found that non adherence to these principles was associated with a small increase in recidivism (r=-0.02), whereas larger decreases were observed with increased adherence to the RNR-principles (two principles, r=0.18; three principles, r=0.26). Since the main objective of forensic treatment is to minimize the risk of reoffending, it is of particular importance to investigate which central eight criminogenic risk factors play a significant role in recidivism among subgroups of violent offenders.
Hence, the central aim of this study was to compare adult male violent offenders diagnosed with SUD (including alcohol) and co-occurring clinical and/or personality disorders (DD-group) with other types of male violent offenders on the strength of the relationship between the central eight risk factors and two outcome measures (recidivism and dropout). Other groups of violent offenders were those with no clinical or personality disorder (No disorders-group), offenders with exclusively Substance Use Disorders (SUD-group), and offenders with one or more comorbid clinical disorders (substance-related disorders excluded) and/or personality disorders (Other disorders-group). Moreover, recidivism and treatment dropout rates were calculated for these subgroups. It was expected that DD-offenders have the highest recidivism and dropout rates and that-across subgroups-the big four criminogenic factors were more predictive of general recidivism than the moderate four criminogenic factors. These factors were expected to be stronger in DD-offenders than other types of violent offenders.
Sample and study protocol
Data were collected prospectively (but retrieved retrospectively) from offenders who were charged with or convicted of a violent offence and who were accepted for a treatment at an outpatient forensic treatment center in The Netherlands between 2008 and 2012. The outpatient forensic treatment center is located in the western part of The Netherlands. Cognitive-behavioral treatment is offered to juvenile and adult offenders with a mental disorder. Following RNR-guidelines, treatment length and intensity are matched to the offenders’ recidivism risk, and treatment modules, amongst which improving coping skills or recognizing and controlling (aggressive) impulses, are tuned to their treatment needs. A number of exclusion criteria for treatment - clinically assessed at the registration and intake phase-are applicable: Offenders who commit offences from a psychosis are referred to a center for general psychiatry or another forensic psychiatric (outpatient) clinic. In addition, severe addiction problems that require supervised detoxification have to be addressed prior to forensic treatment. Clients enter treatment on a voluntary or mandatory base. Voluntary treatment indicates that clients enter treatment on their own initiative, on referral of a general practitioner or another mental health care institute. Mandatory treatment implies that treatment is imposed by a judge. In these cases a probation officer fulfils the supervisory role.
At intake, patients are informed by the therapist about the data collection from electronic patient files, questionnaires, and risk assessments during their treatment, as well as how these data will be used for scientific purposes and to match their treatment to their individual problems. Patients also receive a flyer detailing the data collection procedure and patients are asked to sign an informed consent letter when they agreed on the use of their data for scientific research. Participation in the study was voluntary. The procedure falls within the Dutch Data Protection Act (Dutch DPA) and other specific Dutch healthcare laws, which provides legal provisions on how to deal with the privacy of personal information within the context of, among others, mental health services. The Dutch DPA also includes that all patients have the right to withdraw their previous consent at all times during and after treatment.
The initial sample comprised 1,879 adult violent offenders. Excluded were detained offenders (n=424) since most of the criminogenic risk factors apply to offenders living in the community, offenders who did not consented to use their data for scientific purposes (n=22), and female offenders (n=156) since they represent a very small percentage (8%) compared to the male adults in the sample. The average age of the remaining group of 1,471 violent male offenders at treatment start was 30.93 years (SD=10.38, Range 18-72 years). The majority was born in the Netherlands (78%) and 22% abroad with the most frequently found percentages of offenders being born in Surinam (4.3%), the Caribbean (3%), Morocco (3.9%), and Turkey (2.5%), a little over half of the sample entered treatment on a voluntary basis (53.5%). The average treatment length was 39.37 weeks (SD=15.19, Range 0-130 weeks).
Subgroups based on DSM-IV-TR classification
DSM classification: At intake, a diagnosis of a psychiatric disorder was established by a psychologist or psychiatrist based on an unstructured DSM-IV guided interview (DSM-IV-TR; [31]). The intake session lasts approximately 60 minutes and consists of a screening of issues relevant to outpatient forensic care, amongst which the criminal history and index offence, family (situation), education and work. The clinically assessed diagnosis during the intake session is discussed and determined by a multidisciplinary team of a psychiatrist, psychotherapists, and psychologists.
SUDs were diagnosed in 56.4% of the sample. Clinical and personality disorders were present in 83.6% (including SUD) and 49.7% of the total group, respectively. The most common clinical disorders were developmental disorders (26.5%), impulse control disorders (19.9%), and mood disorders (11.9%). Cluster B personality disorders were the most frequently diagnosed disorders in the sample with an antisocial personality disorder (39.6%) and a borderline personality disorder (10.2%) being the most frequently diagnosed. Other clinical and personality disorders were diagnosed in less than 10 percent of the sample. This also accounted for the presence of intellectual disabilities among the offenders under investigation (7.8%). Blind to their criminal history and recidvism status, the first author categorized the violent offenders in four mutually exclusive subgroups:
1. Offenders without any clinical and/or personality disorder (No disorders group, n=142, 9.7%);
2. Offenders with a clinical disorder (SUD excluded) and/or personality disorder (Other disorders group, n=499, 33.9%);
3. Offenders with only a SUD disorder (SUD group, n=120, 8.2%);
4. Offenders with a dual diagnosis, that is SUD with co-occurring clinical and/or personality disorder (DD group, n=710, 48.3%)
Independent Variables (IVs)
Previous convictions: Data from the Judicial Documentation System (JDS) were used to map the criminal history of the clients prior to treatment. The JDS, managed by the Central Judicial Documentation service (CJD), offers an overview of all (legal) persons who come into contact with the Dutch justice concerning the settled and non-settled criminal cases. In the current study, the number of any convictions prior to treatment was included.
Central eight criminogenic risk factors: Items of the Risk Assessment for outpatient Forensic Mental Health-adult version (RAF MH; [32]) were used to operationalize the big four and moderate three criminogenic risk factors. The substance use criminogenic factor was not operationalizing as an independent variable since the substance related disorders were used to classify offenders in one of the four subgroups described previously. The RAF MH is a generic Structured Professional Judgment (SPJ) risk assessment instrument with the aim of assessing recidivism risk of clients referred to forensic outpatient treatment, suitable for any type of index offense. The psychometric qualities of the youth version of the RAF MH (which is largely identical to the RAF MH for adults) were confirmed for inter-rater reliability (ICC=0.78) and predictive validity (AUC=0.77; [33]). The RAF MH consists of 65 risk factors tapping on several general risk domains such as previous and current offenses, substance use, family/marital circumstances, social network, attitude, and risk management. From these domains, 22 items (34%) were used to calculate the big four and moderate three criminogenic factors. These factors are described by, amongst others, Andrews and Bonta [16] and Andrews, Bonta, and Wormith [19] as follows:
• B1 History of antisocial/criminal behavior: Early onset of antisocial and criminal behavior, and continuing involvement in a number and variety of anti-social and criminal acts (α=0.68);
• B2 Antisocial personality pattern: Poor self-control, stimulation seeking, weak problem solving skills, hostility (α=0.70);
• B3 Antisocial cognitions: Attitudes, values and rationalizations supportive of crime, cognitive emotional states of anger, resentment and defiance (α=0.66);
• B4 Antisocial network: Association with friends and acquaintances who engage in criminal behavior and relative lack of prosocial friends and acquaintances (α=0.58);
• M1 Family/Marital circumstances: Low levels of affection, care, cohesiveness in family and romantic relationships (α=54);
• M2 Education/Employment: Low levels of performance and involvement resulting in dissatisfaction and avoidance of them (α=56);
• M3 Leisure pursuits: Lack of involvement in pro-social leisure and recreational pursuits (α=0.71).
Based on these descriptions, the big four (B1-B4) and the moderate three (M1-M3) criminogenic risk factors were operationalize using a summation of the RAF MH items. Table 1 presents a more detailed overview of how these factors were operationalized and what percentage of the information was missing.
Risk Factor
|
Rating Scale
|
% Missing
|
B1. History of antisocial/criminal behavior
|
Previous convictions
|
Num
|
0 |
Escalation in frequency and severity of committed offences
|
0/1/2
|
0 |
Young age first antisocial behavior
|
0/1/2
|
0 |
Ever been detained
|
No/Yes
|
0 |
Prior violations of conditions
|
0/1/2
|
0 |
B2. Antisocial personality pattern
|
Lack of self-insight
|
0/1/2
|
0 |
Impulsivity
|
0/1/2
|
0 |
Inadequate coping skills
|
0/1/2
|
0 |
Anger management issues
|
0/1/2
|
0 |
B3. Antisocial cognitions
|
Cognitions supportive of crime
|
0/1/2
|
0 |
Minimalizing offence behavior
|
0/1/2
|
0 |
B4. Antisocial network
|
Criminal friends/acquaintances
|
No/Yes
|
0.6
|
Non-criminal friends/acquaintances
|
Yes/No
|
0.9
|
M1. Family/Marital circumstances
|
Unstable partner relationships
|
0/1/2
|
0 |
Relationships with family members
|
0/1/2
|
0 |
Relationship with children (not applicable=0)
|
0/1/2
|
4.8
|
M2. Education/Employment
|
Expelled from/dropped out of school
|
No/Yes
|
0 |
Employment instability
|
0/1/2
|
0 |
Performance at work
|
0/1/2
|
2.7
|
Work relationship with colleagues (no colleagues=0)
|
0/1/2
|
3.1
|
Work relationship with manager (owner=0)
|
0/1/2
|
2.7
|
M3. Leisure pursuits
|
Individual leisure activities
|
0/1/2
|
0 |
Leisure activities with others
|
0/1/2
|
0 |
Table 1: Number-average Molecular weight (Mn), Molecular weight distribution (Mw/Mn), hydrodynamic radius (Rh), and ?-potential for the polymers.
aCalculated from Equation (2), bEstimated from 1H NMR, cEstimated from GPC, dEstimated from DLS.
Dependent Variables (DVs)
Recidivism: From the JDS, recidivism data were also collected (reference date: July 2nd 2014). In the current study, recidivism was defined as a new conviction (No/Yes) for any offence (general recidivism). The follow-up period (i.e., the period between the risk assessment date and the reference date) varied from 0.63 to 79.13 months with an average of 40.99 months (SD=20.56), which is approximately 3.5 years.
Dropout: Offenders were categorized as dropouts when treatment was unilateral terminated by the client or the therapist against the initial agreement. This information was retrieved from the electronic patient files.
Strategy for analyses
Prior to the main analyses, multicollinearity between the Independent Variables (IVs) was tested against the criterion of Variance Inflation Factors (VIFs) of 10, above which serious multicollinearity is assumed [34]. VIFs for the included IVs were all below 1.5, indicating no multicollinearity between the predictor variables. Seven variables had missing values ranging from 0.6 to 4.8% (Table 1). Data were missing completely at random, as indicated by Little’s MCAR test, χ2 (187)=201.27, p=0.225, and were imputed using Full Information Maximum Likelihood (FIML, also known as “Raw Maximum Likelihood”). FIML outperforms most common methods of handling missing data, including list or pair wise deletion, and mean replacement [35].
Mardia’s test of multivariate kurtosis showed that the data in the four subgroups met the assumption of multivariate normality with values below the threshold of 5 [36]: No-disorder group=1.77; Other disorders group=1.15; SUD-only group=-0.58; DD-group=-1.76. Since items differed in number of scoring categories, variables were standardized into z-scores.
Multivariate Analysis of Variance (MANOVA) with Bonferroni post hoc test to adjust for type 1 errors, and chi-square analyses were conducted to assess the differences between subgroups in demographic, treatment and criminal characteristics. As suggested by Field [37], omega squared (ω2) effect sizes were reported for the MANOVA results, using the following formula. The effect size guidelines for omega squared are: Small=0.01; medium=0.06; large=0.14.
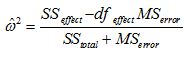
Point-biserial correlations (rpb) were calculated between the dependent and independent variables. Following the guidelines provided by Cohen [38], the strength of the correlation is interpreted as follows: r ≥ 0.10=weak, r ≥ 0.30=moderate, r ≥ 0.50=strong.
Recidivism rates were calculated using Kaplan-Meier survival analysis, which takes into account the individual differences in follow-up periods [39]. The survival analyses were performed with the dates of the first recidivism offense; all subsequent re-offenses were excluded. The log rank test was included to detect significant differences in cumulative recidivism percentages between the subgroups [40].
Multi-group path analysis
In AMOS version 20, a multiple-group structural equation path analysis was performed, using GLS (i.e., Generalized Least Squares) estimation to test whether the criminogenic risk factors had the same effect on general recidivism and treatment dropout across subgroups. Structural Equation Modeling (SEM) has the advantage over conventional regression analyses in SPSS of a simultaneous estimation of co variances between measured constructs, while also testing regression lines of multiple dependent variables. Effects were analyzed using a bootstrap procedure with 95% bias-corrected confidence interval. The bootstrap samples were set at 1,000 as recommended by Cheung and Lau [41] to obtain stable probability estimates. It is considered that if zero is not included on the interval between the lower and the upper bound the effect is statistically significant at p ≤ 0.05 [42]. Figure 1 presents the hypothesized model.
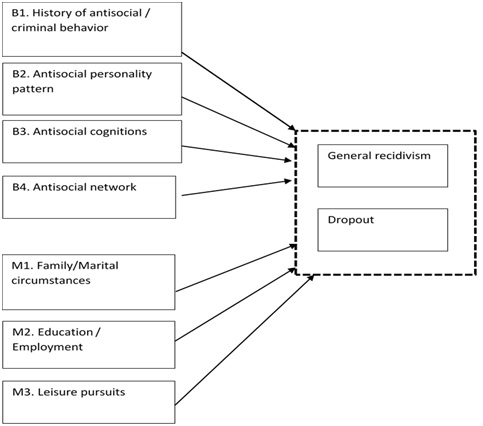
Figure 1: Hypothesized multi-group path model with the big four and moderate three criminogenic factors predictive of both general recidivism and dropout.
Fit indices and thresholds
Following Hu and Bentler [43] and Byrne [36], following selection of fit indices were used to evaluate how well the a priori model reproduced the sample data: Absolute indices chi-square (χ2), the Normed Chi-square (or the chi-square to degrees of freedom ratio, χ2/df), the Root Mean Square Error of Approximation (RMSEA) and the Standardized Root Mean Square Residual (SRMR). The following two incremental fit indices were also included: the Comparative Fit Index (CFI), The Non-Normed Fit Index (NNFI, also known as the Tucker-Lewis Index-TLI). These relative fit indices do not use chi-square in its raw form but compare the chi-square value to a baseline model.
A number of statistic cutoffs for the fit indices are suggested to discriminate between poor, acceptable, and optimal model fit. Although there is no consensus regarding an acceptable χ2/df ratio (CMIN/DF), recommendations range from smaller than 5.0 [44] to preferably around 2.0 [45]. Optimal chi-square values are non-signi?cant. However, signi?cant values do not necessarily indicate poor model at because the chi-square statistic is sensitive to sample size. Therefore, its role in CFA testing for model fit is more descriptive than inferential. For the additional absolute fit indices, a value ≤ 0.06 is needed for the RMSEA index and for SRMR a value close to 0.08 is considered best [43]. Regarding the SRMR, a value close to 0.08 is considered best [43]. Lastly, Kline [42] suggests that for the incremental fit indices CFI and NNFI, values above 0.90 are adequate, although values above 0.95 are more desirable [43].
Sample characteristics
Table 2 presents the demographic and clinical characteristics of the subgroups. At multivariate level significant differences were found between the subgroups for age at start of the treatment, treatment length, and the number of previous convictions, F (9,4401)=9.67, p ≤ 0.001. At univariate level, between groups comparisons revealed a small significant difference for each of the included variables: Age at start of treatment, F (3, 1471)=5.745, p ≤ 0.001, ω2=0.01, treatment length, F (3, 1471)=7.408, p ≤ 0.001, ω2=0.01, and number of previous convictions, F (3, 1471)=15.541, p ≤ 0.001, ω2=0.03. Post hoc multiple comparisons demonstrated that offenders in the Other disorders group were the youngest, offenders in the No disorder group were in treatment for a shorter period of time than offenders from other subgroups, and DD-offenders were convicted more often than offenders from other subgroups. From the chi-square tests results indicated that the number of offenders in mandatory treatment in the DD-group and other disorders group was smaller than those the SUD and No disorders group, χ2 (3)=71.19, p≤0.001. In the No disorder group, the number of offenders with the lowest educational level was smaller compared to the other subgroups, χ2 (6)=13.45, p ≤ 0.036. No significant differences between the subgroups were found for ethnicity, χ2 (3)=7.12, p=0.068
|
DD Group (n=710)
|
SUD Group (n=120)
|
Other Disorders Group (n=499)
|
No Disorders Group (n=142)
|
M
|
SD
|
M
|
SD
|
M
|
SD
|
M
|
SD
|
Age at start treatment
|
30.97
|
9.67
|
29.37
|
10.68
|
32
|
11.12
|
28.31
|
10.32
|
Treatment length (months)
|
40.25
|
14.8
|
38.25
|
16.17
|
39.93
|
15.23
|
33.93
|
15.11
|
#previous convictions
|
9.22
|
11.2
|
7.99
|
12.11
|
6.11
|
7.99
|
4.34
|
4.7
|
|
n
|
%
|
n
|
%
|
n
|
%
|
n
|
%
|
Ethnicity
|
Dutch
|
579
|
81.9
|
98
|
82.4
|
378
|
76.5
|
105
|
75.5
|
Non-dutch
|
126
|
18.1
|
21
|
17.6
|
116
|
23.5
|
34
|
24.5
|
Treatment context
|
Voluntary
|
394
|
55.5
|
47
|
39.2
|
310
|
62.1
|
36
|
25.4
|
Mandatory
|
316
|
44.5
|
73
|
60.8
|
189
|
37.9
|
106
|
74.6
|
Education level*
|
Primary education or less
|
231
|
41.6
|
35
|
37.2
|
154
|
39
|
32
|
29.4
|
Lower secondary
|
298
|
53.7
|
50
|
53.2
|
205
|
51.9
|
67
|
61.5
|
Upper secondary or higher
|
26
|
4.7
|
9
|
9.6
|
36
|
9.1
|
10
|
9.2
|
Table 2: Demographic characteristics, previous convictions and treatment related characteristics of DD-group compared to other subgroups.
* Education levels are derived from the UNESCO’s International Standard Classification of Education (ISCED 2011, UNESCO 2012).
Note: Chi-square differences between groups were considered significant below a p-value of 0.005 due to Bonferroni correction.
Recidivism and dropout rates
The log rank test for general recidivism between the subgroups was significant, χ2 (3)=20.401, p ≤ 0.001. From the cumulative recidivism percentages listed in table 3, it is shown that, DD-offenders and offenders in the SUD-group had the highest recidivism rates (above 50%) compared to the other two subgroups (below 40%). The chi-square test showed a significant difference in dropout rates between the groups, χ2 (3)=23.33, p ≤ 0.001, indicating DD-offenders had the highest dropout rate.
|
DD Group (n=710)
|
SUD Group (n=120)
|
Other Disorders Group (n=499)
|
No Disorders Group (n=142)
|
n
|
%
|
n
|
%
|
n
|
%
|
n
|
%
|
Recidivism
|
427
|
52.3
|
48
|
50.8
|
144
|
39
|
46
|
38.3
|
Dropout
|
346
|
50.8
|
42
|
37.8
|
192
|
40.4
|
45
|
33.1
|
Table 3: General recidivism and dropout rates for subgroups of violent offenders.
Correlations between IVs and DVs
In table 4 point-biserial correlation coefficients are listed between the Independent Variables (IVs) and Dependent Variables (DVs) used in the study. The big four criminogenic risk factors were all significantly related to recidivism and dropout. However, these correlation coefficients were weak. Correlations of moderate strength were found between B1 History of antisocial/criminal behavior and B4 Antisocial network and between B2 Antisocial personality pattern and M1 Mamily/marital circumstances. Other significant correlations between the central eight criminogenic risk factors were weak, indicating minimal contextual overlap between the risk factors.
|
Recidivism
|
Dropout
|
B1
|
B2
|
B3
|
B4
|
M1
|
M2
|
Dropout
|
0.059*
|
|
|
|
|
|
|
|
B1 History of antisocial/criminal behavior
|
0.226***
|
0.167***
|
|
|
|
|
|
|
B2 Antisocial personality pattern
|
0.060*
|
0.200***
|
0.200***
|
|
|
|
|
|
B3 Antisocial cognitions
|
0.126***
|
0.101***
|
0.287***
|
0.265***
|
|
|
|
|
B4 Antisocial network
|
0.141***
|
0.099***
|
0.364***
|
0.198***
|
0.186***
|
|
|
|
M1 Family/Marital circumstances
|
0.03
|
0.155***
|
0.060*
|
0.332***
|
0.102***
|
0.122***
|
|
|
M2 Education/
Employment
|
0.005
|
0.098***
|
0.163***
|
0.232***
|
0.062*
|
0.111***
|
0.101***
|
|
M3 Leisure pursuits
|
0.017
|
0.124***
|
0.170***
|
0.260***
|
0.139***
|
0.153***
|
0.252***
|
0.142***
|
Table 4: Point-biserial correlations (rpb) between IVs and DVs.
Multi-group path analysis
The hypothesized model had an excellent fit to the data, Bollen-Stine bootstrapped χ2=3.68, df=4, p=0.420; CMIN/DF=0.921; RMSEA=0.00, 90% CI=0.000-0.038; SRMR=0.014; CFI=1.00; NNFI=1.02, indicating an overall support for the included predictors of general recidivism and dropout. In table 5 the bias-bootstrapped path estimates (ß), Standardized Errors (SE) and 95% CI for the IVs are presented for each subgroup.
Based on R2 values, the overall model for the DD-group accounted for 4.6% of the variance in general recidivism and 5.1% of the variance in dropout. Compared to the other subgroups these explained variances of recidivism and dropout were in the low range: Recidivism: R2SUD=7.5%; R2No disorders=19.7%; R2Other disorders=9.1%. Dropout: R2SUD=19.7%; R2No disorders=10.9%; R2Other disorders=6.4%).
Except for B1 History of criminal behaviour and M3 Leisure pursuits, different criminogenic factors seemed to contribute to recidivism and dropout in three out of four subgroups of violent offenders. Zooming in on the DD-offenders, recidivism was significantly affected by B1 history of antisocial/criminal behaviour (ß=0.158) and M2 Education/employment (ß=-0.081), indicating that an extended history of criminal behaviour elevated the risk of reoffending, whereas a lower education and/or employment instability decreased this risk. The criminogenic factors significantly related to dropouts in the DD-group were B2 Antisocial personality pattern (ß=0.110), M1 Family/Marital circumstance (ß=0.084), and M3 Leisure pursuits (ß=0.070).
|
DD (N=710)
|
SUD (N=120)
|
Other Disorders (N=499)
|
No Disorders (N=142)
|
Recidivism
|
ß
|
SE
|
95%CI
|
p
|
ß
|
SE
|
95%CI
|
p
|
ß
|
SE
|
95%CI
|
p
|
ß
|
SE
|
95%CI
|
p
|
B1 History of antisocial/ criminal behavior
|
0.158
|
0.041
|
0.069 0.236
|
0.002
|
0.130
|
0.093
|
-0.047 0.310
|
0.162
|
0.195
|
0.048
|
0.101 0.297
|
0.002
|
0.295
|
0.088
|
0.093 0.453
|
0.003
|
B2 Antisocial personality pattern
|
0.048
|
0.039
|
-0.031 0.122
|
0.271
|
-0.254
|
0.113
|
-0.476 -0.039
|
0.029
|
0.018
|
0.049
|
-0.08 0.114
|
0.720
|
0.165
|
0.091
|
-0.017 0.343
|
0.083
|
B3 Antisocial cognitions
|
0.055
|
0.040
|
-0.028 0.135
|
0.163
|
-0.063
|
0.089
|
-0.229 0.113
|
0.535
|
0.111
|
0.048
|
0.014 0.197
|
0.030
|
0.037
|
0.081
|
-0.121 0.203
|
0.573
|
B4 Antisocial network
|
0.041
|
0.038
|
-0.036 0.111
|
0.292
|
0.086
|
0.088
|
-0.088 0.257
|
0.338
|
0.071
|
0.048
|
-0.025 0.166
|
0.137
|
0.105
|
0.088
|
-0.069 0.272
|
0.272
|
M1 Family/Marital circumstances
|
-0.025
|
0.040
|
-0.105 0.052
|
0.531
|
0.016
|
0.107
|
-0.205 0.219
|
0.918
|
-0.055
|
0.045
|
-0.140 0.038
|
0.245
|
-0.265
|
0.075
|
-0.404 -0.112
|
0.004
|
M2 Education
/Employment
|
-0.081
|
0.034
|
-0.147 -0.012
|
0.026
|
0.116
|
0.099
|
-0.069 0.322
|
0.240
|
0.028
|
0.042
|
-0.051 0.114
|
0.485
|
0.027
|
0.079
|
-0.176 0.135
|
0.754
|
M3 Leisure pursuits
|
0
|
0.039
|
-0.082 0.074
|
0.959
|
0.018
|
0.107
|
-0.196 0.225
|
0.856
|
-0.028
|
0.049
|
-0.120 0.072
|
0.546
|
-0.168
|
0.094
|
-0.370 0.007
|
0.059
|
Dropout
|
B1 History of antisocial/criminal behavior
|
0.052
|
0.040
|
-0.027 0.138
|
0.163
|
0.147
|
0.090
|
-0.026 0.323
|
0.110
|
0.202
|
0.049
|
0.104 0.302
|
0.003
|
0.064
|
0.090
|
-0.114 0.235
|
0.524
|
B2 Antisocial personality pattern
|
0.110
|
0.039
|
0.030 0.184
|
0.006
|
0.066
|
0.109
|
-0.158 0.265
|
0.603
|
0.092
|
0.048
|
-0.004 0.199
|
0.058
|
0.201
|
0.101
|
0.020 0.379
|
0.068
|
B3 Antisocial cognitions
|
0.036
|
0.039
|
-0.044 0.107
|
0.418
|
0.028
|
0.095
|
-0.162 0.212
|
0.785
|
-0.001
|
0.050
|
-0.094 0.090
|
0.991
|
-0.015
|
0.088
|
-0.186 0.169
|
0.881
|
B4 Antisocial network
|
0.031
|
0.039
|
-0.046 0.110
|
0.400
|
0.067
|
0.094
|
-0.245 0.116
|
0.457
|
-0.55
|
0.050
|
-0.147 0.041
|
0.311
|
0.070
|
0.087
|
-0.102 0.243
|
0.374
|
M1 Family/Marital circumstances
|
0.084
|
0.040
|
0.003 0.168
|
0.040
|
0.200
|
0.116
|
0.039 0.411
|
0.104
|
0.093
|
0.050
|
-0.010 0.191
|
0.065
|
0.039
|
0.101
|
-0.152 0.241
|
0.706
|
M2 Education/
Employment
|
0.038
|
0.037
|
-0.036 0.114
|
0.312
|
0.007
|
0.093
|
-0.197 0.169
|
0.996
|
0.021
|
0.047
|
-0.072 0.114
|
0.681
|
0.166
|
0.100
|
-0.048 0.353
|
0.115
|
M3 Leisure pursuits
|
0.070
|
0.038
|
-0.001 0.144
|
0.052
|
0.242
|
0.100
|
0.043 0.433
|
0.022
|
0.012
|
0.049
|
-0.088 0.103
|
0.842
|
-0.249
|
0.094
|
-0.426 -0.046
|
0.017
|
Table 5: Multi-group path analysis: bias-corrected bootstrap path estimates (ß), SEs and 95% CIs for IVs as predictors of recidivism and dropout for subgroups of violent offenders.